Research
Stratigraphic correlation
Correlating stratigraphic signals (e.g., geophysical well logs) is a fundamental task in sedimentology and stratigraphy, both in industry and academia. We have developed a Python package called 'ChronoLog' that relies on 3D optimization of a large number of pairwise stratigraphic correlations to build a chronostratigraphic framework that is consistent in three dimensions. The pairwise correlations use the dynamic time warping (DTW) algorithm. In collaboration with USGS scientists, we have also applied the DTW approach to testing whether turbidites along the Cascadia subduction zone - potential records of megathrust earthquakes - can be correlated over large distances.Basin Research GSA Bulletin
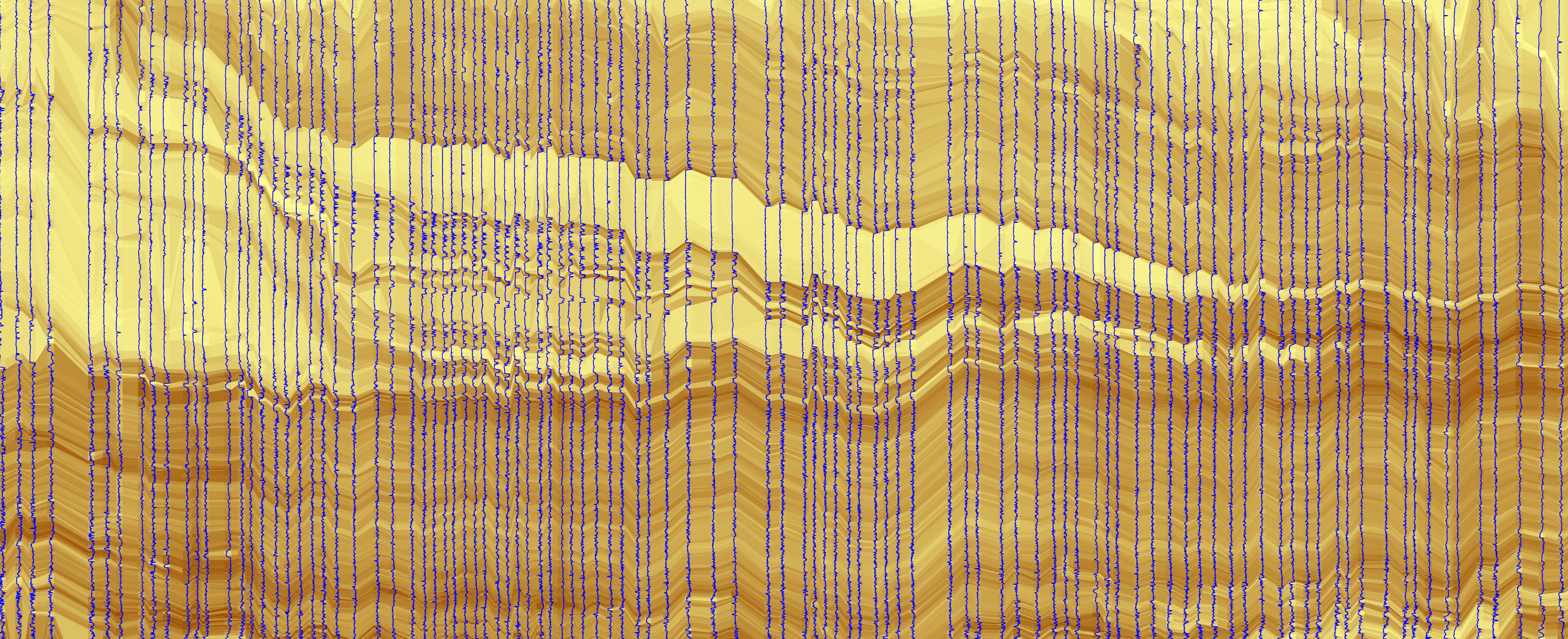
The geomorphology and stratigraphy of meandering channels
During the last few years, our group has spent significant time working on the morphology and stratigraphy of meandering / sinuous channels. The focus was initially on submarine channels and largely based on three-dimensional seismic data and simple kinematic modeling. However, I think that development of sinuosity in submarine channels shares similarities with meandering rivers, and a lot remains to be learned from trying to apply our knowledge of fluvial systems in the submarine realm. In addition, there are many simple aspects of fluvial meandering models that may be well known to geomorphologists but have been not fully explored yet in terms of impact on stratigraphy.We have also explored the idea that the curvature of meandering channels may be a good predictor of migration rate, contrary to the common belief that the relationship between the two is higly nonlinear and noisy; and have shown that meandering rivers that are not too affected by variations in erodibility may be well described by simple curvature-based models of meandering.
Geological Society Geological Society The Depositional Record Geosphere GSA Bulletin Geology Geology SEPM MPG
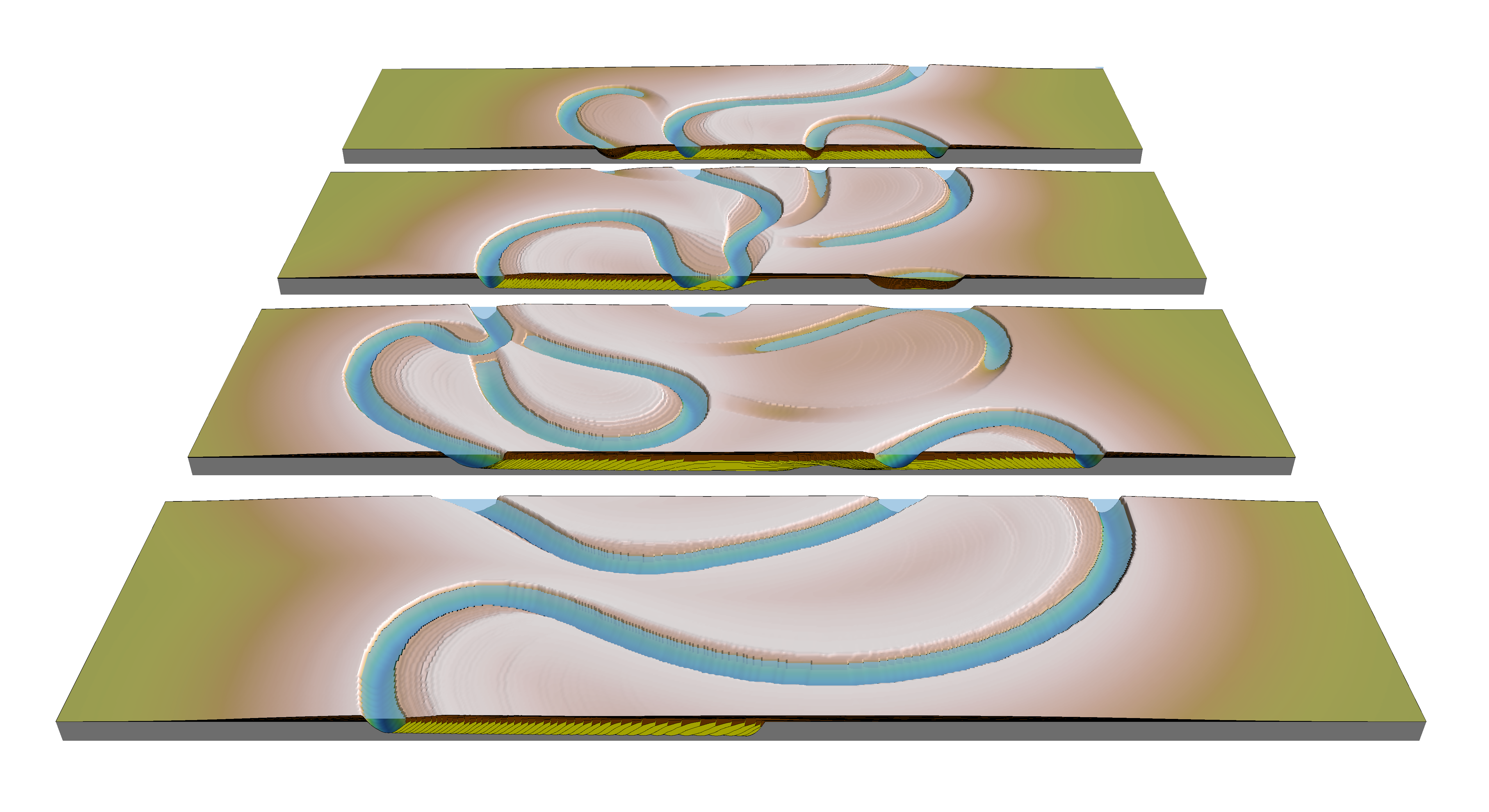
AI/ML applications in the geosciences
During the last few years, I have been co-teaching a course (with Sergey Fomel) on 'Machine Learning Applications in the Geosciences'. In parallel with the course, we worked on a few projects that focus on the use of image segmentation to solve various geoscience problems. One of the outcomes of this work is an open-source Python package called 'segmenteverygrain', which is designed to segment images of all kinds of grains, including thin sections photographed in transmitted light, sand grains in reflected light, gravel and boulders on beaches and river bars or outcrops. 'segmenteverygrain' is based on an initial Unet model that has been trained on a variety of images of grains; the output of this model is passed on to Meta's 'Segment Anything' model, which is a more general-purpose segmentation algorithm.GitHub
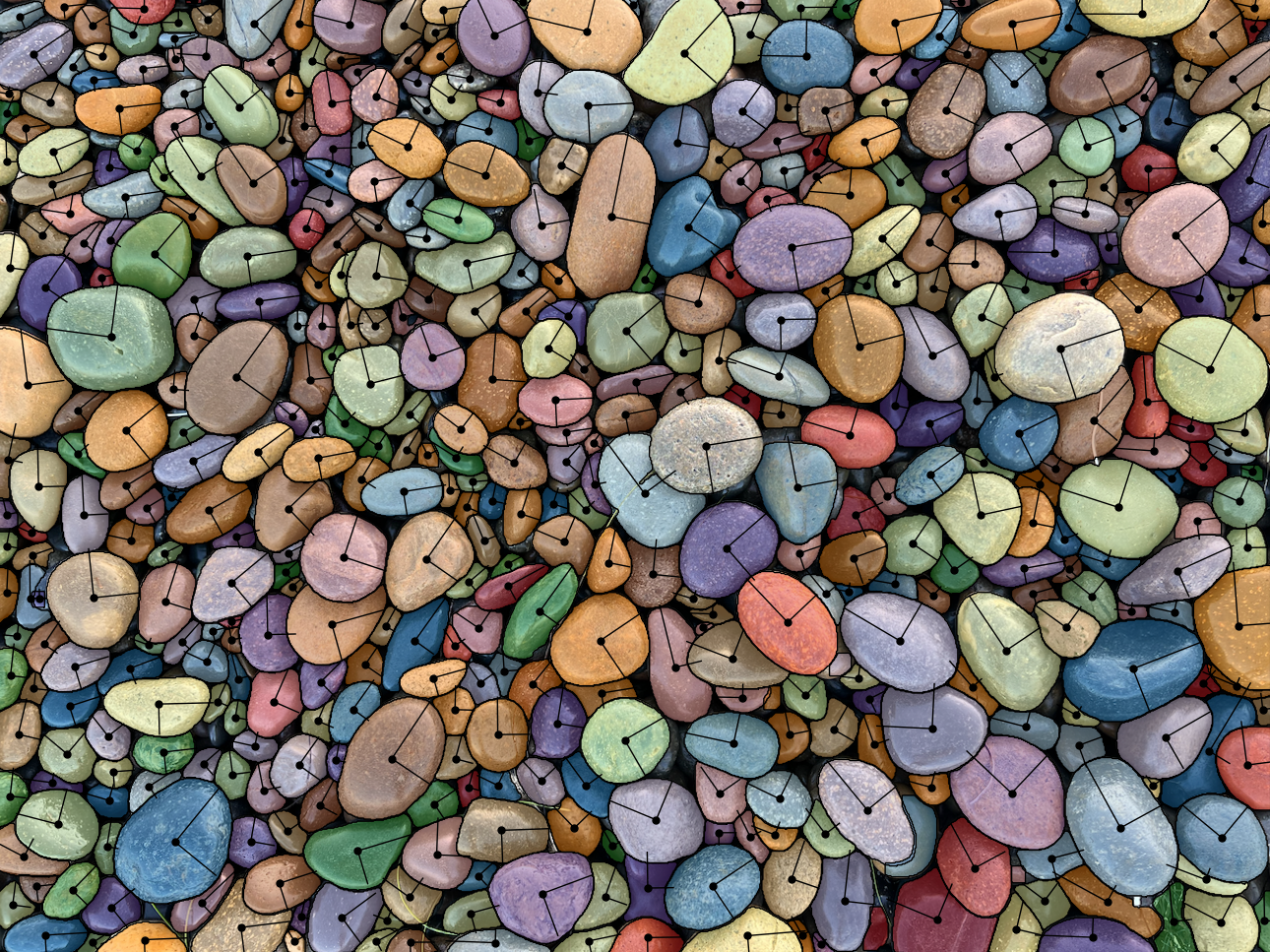
Seismic stratigraphy of deepwater systems
Three-dimensional seismic reflection data has been called the "geological Hubble", and for a good reason. Marine seismic data tends to be of high quality and there is an increasing number of datasets that are becoming publicly available. Early on in my career, I have spent significant time interpreting seismic reflection data, and I find that this kind of data is unparalleled when it comes to understanding the true three-dimensionality and spatial context of depositional systems. The image below is from two channel-levee systems (Fuji and Einstein) in the Eastern Gulf of Mexico (from Sylvester et al., 2012, SEPM Special Publication).SEPM Springer
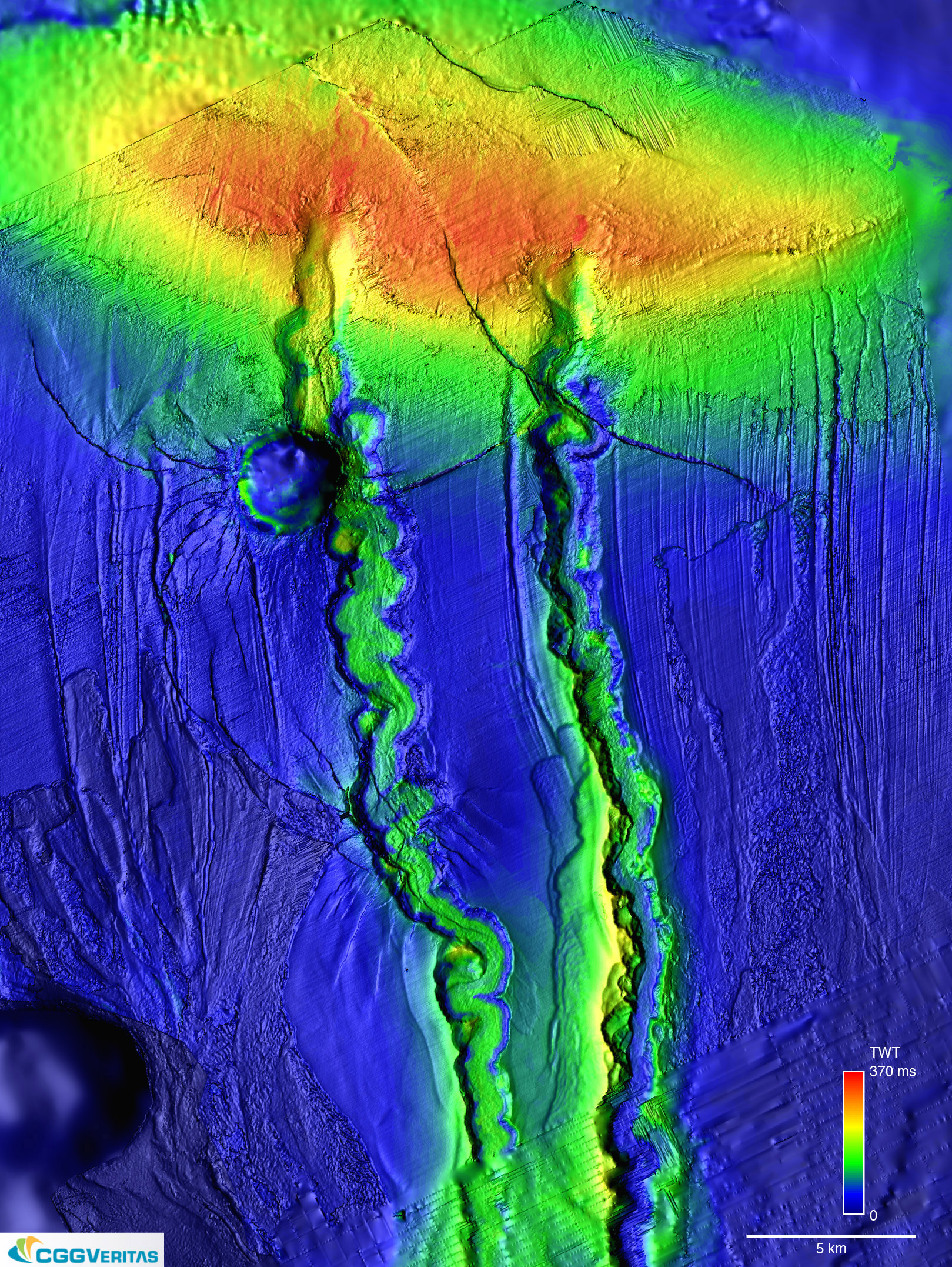